之前介绍了使用maftools | 从头开始绘制发表级oncoplot(瀑布图) R-maftools包绘制组学突变结果(MAF)的oncoplot或者叫“瀑布图”,以及一些细节的更改和注释。 本文继续介绍maftools对于MAF文件的其他应用,为更易理解和重现,本次使用TCGA下载的LIHC数据。 一 数据部分setwd("C:\\Users\\Maojie\\Desktop\\maftools-V2\\") library(maftools) laml.maf = read.csv("TCGA.LIHC.mutect.maf.csv",header=TRUE)
#本次只展示maf的一些统计绘图,只读入组学数据,不添加临床数据 laml = read.maf(maf = laml.maf) #查看数据的基本情况 laml An object of class MAF ID summary Mean Median 1: NCBI_Build 1 NA NA 2: Center 1 NA NA 3: Samples 364 NA NA 4: nGenes 12704 NA NA 5: Frame_Shift_Del 1413 3.893 3 6: Frame_Shift_Ins 551 1.518 1 7: In_Frame_Del 277 0.763 0 8: In_Frame_Ins 112 0.309 0 9: Missense_Mutation 28304 77.972 63 10: Nonsense_Mutation 1883 5.187 4 11: Nonstop_Mutation 45 0.124 0 12: Splice_Site 1051 2.895 2 13: Translation_Start_Site 65 0.179 0 14: total 33701 92.840 75
可以将MAF文件的gene ,sample的 summary 的信息,输出到laml前缀的summary文件 write.mafSummary(maf = laml, basename = 'laml') laml_geneSummary.txt 
laml_sampleSummary.txt 
二 绘图部分1,首先绘制MAF文件的整体结果图plotmafSummary(maf = laml, rmOutlier = TRUE, addStat = 'median', dashboard = TRUE, titvRaw = FALSE) 
2,oncoplot图#oncoplot for top ten mutated genes. oncoplot(maf = laml, top = 20) 
添加SCNA信息,添加P值信息,添加临床注释信息,更改颜色等可参考 链接 。。 3 Oncostrip可以使用 oncostrip 函数展示特定基因在样本中的突变情况,此处查看肝癌中关注较多的'TP53','CTNNB1', 'ARID1A'三个基因,如下: oncostrip(maf = laml, genes = c('TP53','CTNNB1', 'ARID1A')) 
4 Transition , Transversionstitv函数将SNP分类为Transitions_vs_Transversions,并以各种方式返回汇总表的列表。汇总数据也可以显示为一个箱线图,显示六种不同转换的总体分布,并作为堆积条形图显示每个样本中的转换比例。 laml.titv = titv(maf = laml, plot = FALSE, useSyn = TRUE) #plot titv summary plotTiTv(res = laml.titv) 
5 Rainfall plots使用rainfallPlot 参数绘制rainfall plots,展示超突变的基因组区域。detectChangePoints设置为TRUE,rainfall plots可以突出显示潜在变化的区域. brca <- system.file("extdata", "brca.maf.gz", package = "maftools") brca = read.maf(maf = brca, verbose = FALSE) rainfallPlot(maf = laml, detectChangePoints = TRUE, pointSize = 0.6) 
6 Compare mutation load against TCGA cohorts通过tcgaComapre 函数实现laml(自有群体)与TCGA中已有的33个癌种队列的突变负载情况的比较。 #cohortName 给输入的队列命名 laml.mutload = tcgaCompare(maf = laml, cohortName = 'LIHC-2') 
7 Genecloud使用 geneCloud 参数绘制基因云,每个基因的大小与它突变的样本总数成正比。 geneCloud(input = laml, minMut = 15) 
8 Somatic Interactions癌症中的许多引起疾病的基因共同发生或在其突变模式中显示出强烈的排他性。可以使用somaticInteractions 函数使用配对Fisher 's精确检验来分析突变基因之间的的co-occurring 或者exclusiveness。 #exclusive/co-occurance event analysis on top 10 mutated genes. Interact <- somaticInteractions(maf = laml, top = 25, pvalue = c(0.05, 0.1)) #提取P值结果 Interact$gene_sets gene_set pvalue 1: CTNNB1, AXIN1, TP53 0.0001486912 2: CTNNB1, TP53, ARID1A 0.0018338597 3: AXIN1, TP53, APOB 0.0087076043 4: CSMD3, AXIN1, ALB 0.0130219628 5: AXIN1, TP53, ALB 0.0173199619 6: CTNNB1, AXIN1, ARID1A 0.0363739468

可以看到TP53和CTNNB1之间有较强的exclusiveness,也与文献中的结论一致。 9 Comparing two cohorts (MAFs)由于癌症的突变模式各不相同,因此可是 mafComapre 参数比较两个不同队列的差异突变基因,检验方式为fisher检验。 #输入另一个 MAF 文件 Our_maf <- read.csv("Our_maf.csv",header=TRUE) our_maf = read.maf(maf = Our_maf)
#Considering only genes which are mutated in at-least in 5 samples in one of the cohort to avoid bias due to genes mutated in single sample. pt.vs.rt <- mafCompare(m1 = laml, m2 = our_maf, m1Name = 'LIHC', m2Name = 'OUR', minMut = 5) print(pt.vs.rt) 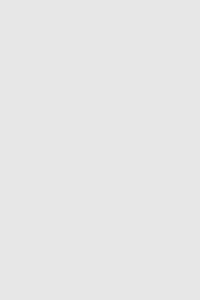
1) Forest plots比较结果绘制森林图 forestPlot(mafCompareRes = pt.vs.rt, pVal = 0.01, color = c('royalblue', 'maroon'), geneFontSize = 0.8) 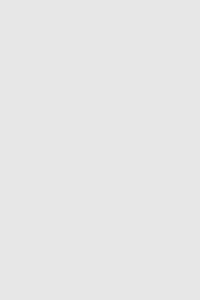
10 Oncogenic 信号通路`OncogenicPathways 功能查看显著富集通路
OncogenicPathways(maf = laml) #会输出统计结果 Pathway alteration fractions Pathway N n_affected_genes fraction_affected 1: RTK-RAS 85 68 0.8000000 2: WNT 68 55 0.8088235 3: NOTCH 71 52 0.7323944 4: Hippo 38 30 0.7894737 5: PI3K 29 24 0.8275862
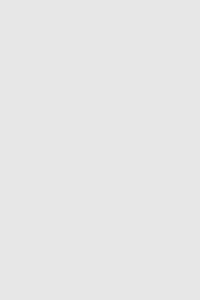
可以对上面富集的通路中选择感兴趣的进行完成的突变展示: PlotOncogenicPathways(maf = laml, pathways = "PI3K") 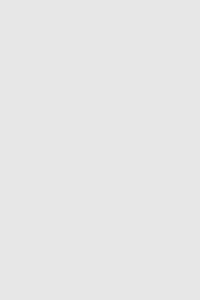
好了,以上就是使用maftools包对MAF格式的组学数据的汇总,分析,可视化。
|